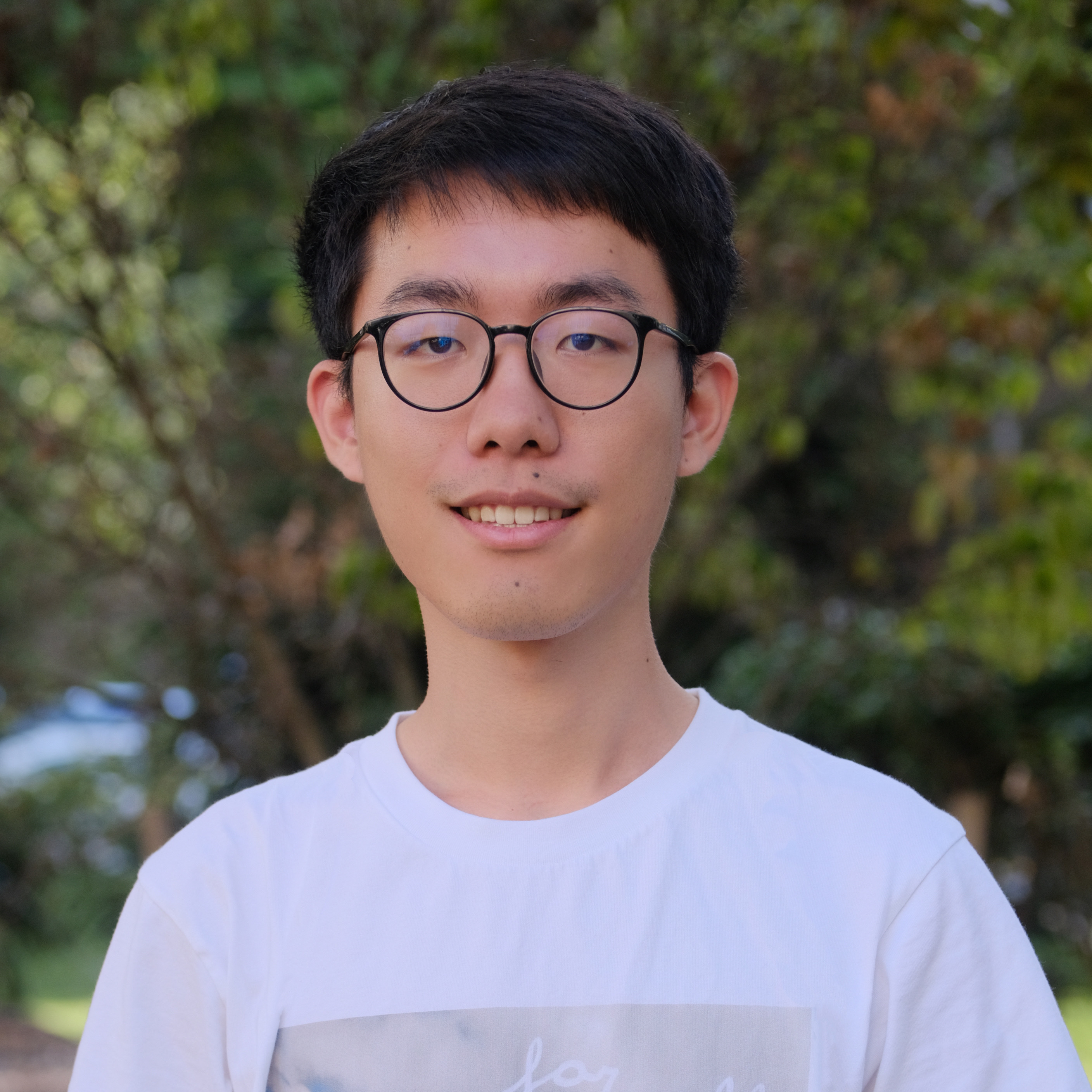
Hi! I am Muzhe (沐哲, pronounced /mù tʂɤ́/), currently a visiting research student at the Human-Computer Interaction Institute (HCII) at Carnegie Mellon University (CMU) advised by David Lindlbauer.
My research interest lies at the intersection of Human-Computer Interaction, eXtended Reality, and Learning Sciences. I investigate human cognitive processes (e.g., learning, attention, agency) in relation to input-output channels (e.g., vision, hearing, motor systems) and develop interfaces / interaction techniques / experiences to augment them.
I recently graduated from Master’s in Educational Technology and Applied Learning Science at CMU HCII. I did my Bachelor’s in Computer Science at the University of Michigan (UMich) and Electrical and Computer Engineering from Shanghai Jiao Tong University. I was fortunate to kick off my research journey at UMich with Anhong Guo and Xu Wang building AR-based intelligent tutoring systems, and during my Master’s at CMU HCII, also collaborated with Hong Shen on socio-technical responsible AI.
Outside of work, I enjoy sports ⛸️ 🏀, music 🎤 🎹, and travel 🏞️.
News
-
Feb 2025
I've decided to return to UMich for my PhD! Grateful to everyone who chatted with me and shared opportunities.
-
Oct 2024
Had a fruitful two weeks attending UIST and ISMAR and presenting our work New Ears.
-
Jul 2024
Finished my master's program at CMU HCII.